
Biography
Prof. Dr. Clara Viñas Teixidor graduated in Chemistry at the Universitat Autònoma de Barcelona and later in Pharmacy at the Universitat de Barcelona. She worked as a pre-doctoral student at the Prof. Rudolph’s laboratory at The University of Michigan for a year. She is a Research Professor at the Institut de Ciència de Materials de Barcelona that belongs to the Spanish Council for Scientific Research since 2006. Previously, she worked in an industry dedicated to recovery of industrial residual waters, and at public institution involved in food science analysis as well as environmental control.
Her fields of research involve synthesis and derivatisation of boron clusters to be applied in medicine and biosensors, among others.
PAQ-Collabora Project
Development of New kit for Latent Fingerprint detection and Authentication "KIDAEM"
PAQ-Collabora Project
Development of New kit for Latent Fingerprint detection and Authentication "KIDAEM"
PAQ-Collabora Project
Development of New kit for Latent Fingerprint detection and Authentication "KIDAEM"
PROJECT PARTNERS
GEOGLOB-Lab Faculty of Sciences of Sfax
​
LATIS-Lab National School of Engineers of Sousse
CEM-Lab National School of Engineers of Sfax
SOGIMEL Private Company
​
PROJECT PARTNERS
GEOGLOB-Lab Faculty of Sciences of Sfax
​
LATIS-Lab National School of Engineers of Sousse
CEM-Lab National School of Engineers of Sfax
SOGIMEL Private Company
​
RESUME
RESUME
Registration
PROJECT NEWS
​
04/04/2019 Call for POSTDOC recrutment
30/03/2019 Signature of the financial support memorandum by the minister of HER
22/03/2019 Coaching session @ Ministry
01/03/2019 First meeting of project members
​
​
Miss.Rihab Gargouri (PhD Student)
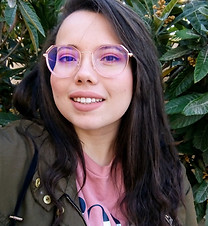
Resume
​
Recently, accurate predictions using machine learning (ML) algorithms have been mentioned in several fields. In this study, we constructed two quantitative structure-property relationships (QSPR) models by a dataset of organic compounds (small molecules) having available experimental heat of vaporization ) data, to predict the heat of vaporization of polymer repeat units. For that, Multiple Linear Regression (MLR) and Kernel Ridge Regression (KRR) models were created. Thirteen workable parameters (descriptors) were involved in both ML models, including count descriptors and quantum chemical descriptors, and calculated by different approaches from both small molecules and repeat unit structure. The dataset of small molecules was randomly divided into a training sample (70%) and a testing sample (30%). MLR demonstrated the best performance for outcome prediction for polymer repeat units with a correlation coefficient (R2 ) of 0.835, a MAE of 3.769 (kJ/mol), and a RMSE of 4.531 (kJ/mol). Our results indicated that most of the predicted values are in good agreement with the experimental data.
​
Publications
Conference Certificates


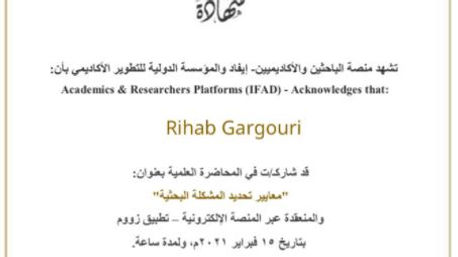

Course Certificates

